The low predictability of agricultural cycles in semi-arid regions
The low predictability of agricultural cycles in semi-arid regions [1].
In the early 2010s, chaotic attractors were captured for the cycles of cereal crops in North Morocco. This result was unexpected for several reasons. It was the first time a chaotic attractor was obtained from remote sensing data. It was also the the first « weakly dissipative » dynamics directly captured from observational data. Moreover, its dynamics was produced by a canonical form with which only highly dissipative dynamics had been produced before. Was it a hapax? Or was there any generality in this result?
To obtain a chaotic attractor is often an important result because it shows that the low predictability — a comon feature of environmental dynamics — is not just a problem of natural hazard, that is, of probability. Indeed, chaos is — deterministic — which means that the state of the system at a given time entirely determines its state immediately after. To obtain a chaotic attractor from observational data gives a strong argument for determinism, and therefore, for a strong order hidden behind the low predictability.
The theory of chaos is particularly well designed to study dynamics of low predictability. It enables an alternative way to do science [2]. Instead of trying to solve equations (which are not always known) as mathematicians commonly do, this theory fosters the use of a geometric space enabling to follow the evolution in time of the studied system state. Since this space can be reconstructed directly from observational data, it can be used to study dynamics under real world conditions. Moreover, this space being independent from the initial conditions (because it contains them all) it can be used to unveil the underlying equations governing the observed dynamics, or to get compact approximation of them. Other approaches have been developped, but very few of them were able to extract chaotic attractors from environmental or experimental time series.
First results obtained for semi-arid region in 2011
In 2011, a chaotic attractor was obtained for the cycles of cereal crops in North Morocco [3]. A time series of vegetation index observed by remote sensing was used for this purpose. A chaotic attractor is an oriented trajectory in this state space. It is characterized by specific properties. It takes place in a bounded part of this space and it is non-periodic: it is never looping back to itself, even after numerous loops (otherwise, it would be fully predictable) [4]. Such an attractor is also characterized by diverging property giving rise to unpredictability: two states taken close one to another in the phase space will diverge. To remain in a bounded space, its structure must present folding, and possibly tearing properties. Finally, the geometry of the attractor should also be fractal, that is, it should be auto-similar by scale change. All these properties were confirmed for the first chaotic attractors obtained for cereal crops from remote sensing data: non-periodic and bounded trajectory, divergence of the flow (characterized by a positive first Lyapunov exponent), with folding and characterized by a fractal dimension (D = 2.68 and D = 2.75).
To find a fractal dimension was coherent for cereal crops cycles, which yields are known to be difficult to foresee. However, the attractor obtained for crops cycles was different from what most usual chaotic attractors. Indeed, most of the well known chaotic attractors, such as the Lorenz [5] or the Rössler [6] attractors arise from highly dissipative systems. As a consequence, although developed in three dimensions, these attractors are flat (locally bidimensional) almost everywhere and their fractal dimension is close to two (respectively 2.05 and 2.06 for usual parameter values). Surprisingly, the first cereal crops attractor was not flat at all (see for example Fig. 1, the cross section of the chaotic attractor obtained), and its fractal dimension was close to three which means that divergence and convergence speeds were of similar amplitude: the attractor was belonging to weakly dissipative chaos.
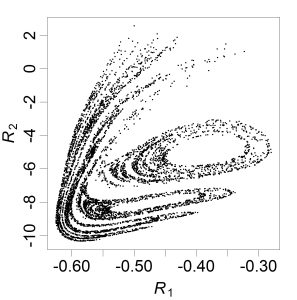
Weakly dissipative chaos is rather rare in dynamical systems and it was the first time this type of dynamics could be extracted directly from observational data. Previous cases were weakly dissipative chaos obtained by Langford [7] or by Lorenz [8] in the early 1980s, but these were derived from theoretical considerations, not from observational data. This result has provided a strong argument for determinism hidden behind the low predictability of the agricultural cycles from the real world. But, this result obtained in 2011 was local, since obtained from a single time series and therefore specific to to chosen location. The generality of this result was thus questionable.
New analyses applied to four other locations
To investigate this question, the global modelling technique was applied again to four other provinces in Morocco: two ones located on the coastal area (El Jadida and Safi provinces), two others located inland (Khourigba and Khenifra). The global modelling technique aims to otbain equations directly from observational time series, without strong hypotheses. Even when applied to a single variable, the approach can be applied to multiple time series or to a single time series with gaps (see [9]). The GPoM package [10] was used for this purpose.
In the analyses just published in the Journal of Difference Equations and Applications [1], time series of Normalized Difference Vegetation Index (NDVI) from AVHRR sensors were considered. The provinces were analysed one by one, two by two and the four ones altogether, either in association (concatenated time series), or in aggregation (averaged time series). Models could be obtained and validated in numerous cases. One example, obtained from the time series of NDVI spatially averaged on the four provinces, is provided in Figure 2.

All the properties of chaos were confirmed in most of the cases, that is: deterministic and characterized by a non-periodic, diverging, bounded and fractal attractor. Weakly dissipative chaos was also confirmed in all these cases (2.28 ≤ D ≤ 2.80) except one (for which dimension was a bit lower, D = 2.10).
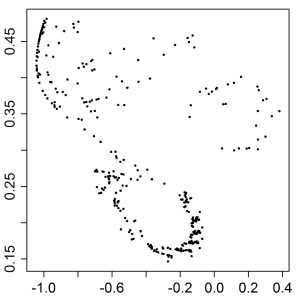
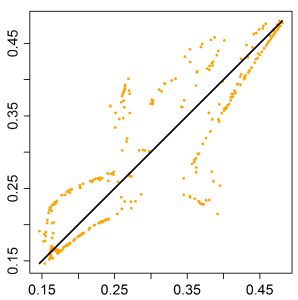
Weakly dissipative chaos was thus confirmed for the cycles of cereal crops in semi-arid region. How to explain such a type of dynamics? Their interpretation is challenging. It is obvious that energy, water, and nutrients are required to grow plants. However, the analysis being based on a single variable used to monitor the cycle of cereal crops – here the vegetation index measured from remote sensing – as a whole. What is characterized, here, is the global dynamics of the plants and its agricultural cycle. It tells us that the plant dynamics, as it is observed from the point of view of the vegetation index, is close to optimal. In other words, the chosen plants as well as the agricultural practice, are well adapted to the meteorological and hydric conditions of the soil, at the provinces scale.
Author
This post was written by Sylvain Mangiarotti at CESBIO
References:
2022. Chaotic attractors captured from remote sensing time series for the dynamics of cereal crops, Journal of Difference Equations and Applications, DOI: 10.1080/10236198.2022.2152336
Mangiarotti, 2021. Chaos : from theory to applications for the 80th birthday of Otto E. Rossler, Chaos, 2021, 31(6), 060402. https://doi.org/10.1063/5.0058332
C. Letellier C., L.F. Olsen, S.
R. Lozi, “Giga-periodic orbits for weakly coupled tent and logistic discretized maps,” in Proceedings of International Conference on Industrial and Applied Mathematics, Modern Mathematical Models: Methods and Algorithms for Real World Systems, 4–6 December 2004, edited by A. H. Siddiqi, I. S. Duff, and O. Christensen (Anamaya, New Delhi, 2004).
[5] E.N. Lorenz. 1963. Deterministic nonperiodic flow, Journal of Atmospheric Science, 20(2), 130-141. <a href="https://doi.org/10.1175/1520-0469(1963)0202.0.CO;2″>https://doi.org/10.1175/1520-0469(1963)020<0130:DNF>2.0.CO;2
[6] O.E. Rössler, 1976. An equation for continuous chaos. Physics Letters A, 1976, 31, 259-264. https://doi.org/10.1016/0375-9601(76)90101-8
[7] W.F. Langford, 1984. Numerical studies of torus bifurcations, International Series of Numerical Mathematics, 70, 285–295. DOI: 10.1007/978-3-0348-6256-1_19.
[8] E.N. Lorenz, 1984. Irregularity: A fundamental property of the atmosphere, Tellus, 36(A), 98–110. https://doi.org/10.1111/j.1600-0870.1984.tb00230.x
[9] S. Mangiarotti, F. Le Jean, M. Huc, C. Letellier, 2016. Global modeling of aggregated and associated chaotic dynamics, Chaos, Solitons & Fractals, 83. 82-96. https://doi.org/10.1016/j.chaos.2015.11.031
[10] S. Mangiarotti, M. Huc, F. Le Jean, M. Chassan, L. Drapeau [ctb], GPoM: Generalized Polynomial Modelling. Version 1.3, CeCILL-2 licence. https://CRAN.R-project.org/package=GPoM